XNLG
XNLG stands for “Cross-lingual Natural Language Generation” which is an encoder-decoder cross-lingual model designed for Natural Language Generation (NLG) tasks such as question generation and abstractive summarization. This model was created by Microsoft in 2019 and published in their paper: Cross-Lingual Natural Language Generation via Pre-Training. The official code for this paper is found in the following GitHub repository: xnlg.
As shown in the following figure, XNLG is an encoder-decoder Transformer model pre-trained on on monolingual NLG training data and can be fine-tuned later on other languages which can boost performance for the low-resource settings.
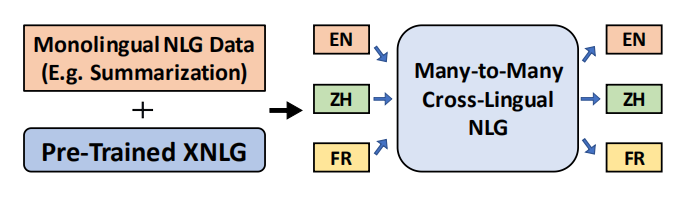
Pre-training
Given a parallel corpus $\mathcal{D}_{p}$ and a monolingual corpus $\mathcal{D}_{m}$, the XNLG pre-training is done in two stages as shown below:
- Stage #1: pre-trains the encoder on two tasks: Masked Language Modeling (MLM) and cross-lingual Masked Language Modeling (XMLM) where the encoder learns to minimize:
- Stage #2: pre-trains the model where the decoder parameters are updated while freezing the encoder parameters on two tasks: (Denoising Auto-Encoding) DAE and cross-lingual Auto-Encoding (XAE). Here, the model learns to minimize:
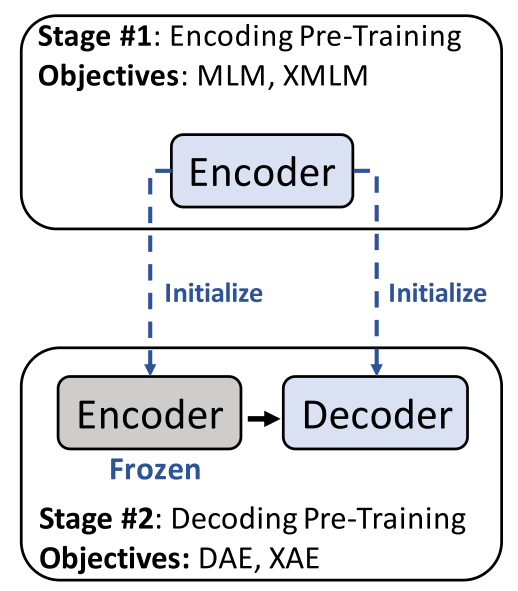
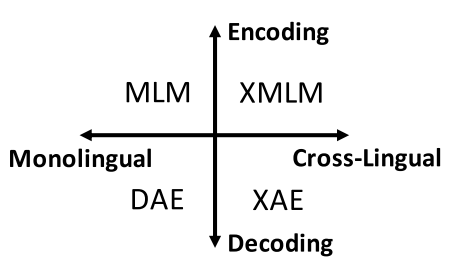
These are the the two stages of pre-training in XNLG, now let’s dive deeper into pre-training tasks mentioned above:
- Masked Language Modeling (MLM):
The masked language modeling (MLM) task was first proposed by the BERT paper. Given an input sequenc $x$, MLM randomly masks $15\%$ of the tokens in a monolingual sentence. Each masked token is substituted with a special token $\left\lbrack M \right\rbrack$, a random token, or the unchanged token with probabilities of $80\%$, $10\%$, and $10\%$, respectively to create a masked sequence $x_{\backslash M_{x}}$ knowing that $M_{x}$ is the set of randomly masked positions. The monolingual MLM loss is defined as:
- Cross-lingual Masked Language Modeling (XMLM):
Similar to MLM, the masked token prediction task can be extended to cross-lingual settings. Given a parallel corpus, bilingual sentences $\left( x,\ y \right)$ can be concatenated to form one sequence which can be used as the input of MLM. Given $M_{x}$ and $M_{y}$ as the sets of masked positions of $x$ and $y$ respectively, the XMLM loss is defined as:
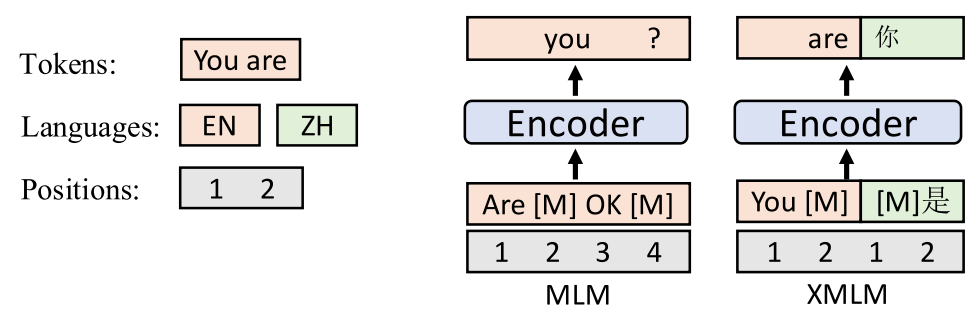
- Denoising Auto-Encoding (DAE):
Given monolingual sentence $x$, DAE applies noise functions over the input sentence producing perturbed sentence $\widehat{x}$. Its objective is to train the whole model to restore the original sentence. The noise functions applied here are: shuffling, randomly dropping tokens with a probability of $0.1$, and randomly replacing tokens with the special padding token [P] with a probability of $0.1$. The DAE loss function is defined as:
- Cross-Lingual Auto-Encoding (XAE):
XAE is a the multilingual-version DAE which can be viewed as a machine translation task. The cross-lingual auto-encoding loss is:
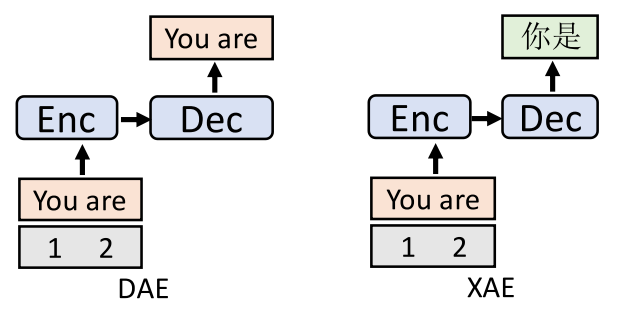
Fine-tuning
In the fine-tuning procedure, there are two scenarios:
-
Fine-tuning for Any-to-Others NLG:
Fine-tuning any language to non-English. In this scenario, they observed catastrophic forgetting of target language. To overcome that, they keep the decoder and the word embeddings frozen and only update the encoder parameters during fine-tuning. -
Fine-tuning for Any-to-English NLG:
Fine-tuning any language to English. In this scenario, they freeze the encoder parameters, and update the decoder parameters.
Note:
When the target language is the same as the language of training data, they fine-tuned all parameters.
Experiments
They conducted experiments over two cross-lingual NLG downstream tasks: cross-lingual question generation, and cross-lingual abstractive summarization. They used a pre-trained XNLG with a 10-layer encoder and a 6-layer decoder. For every Transformer layer, they used $1024$ hidden units, $8$ attention heads, and GELU activations.
In the first pre-training stage, they directly used the pre-trained XLM to initialize the XNLG parameters. In the second pre-training stage, they used Wikipedia as the monolingual data for the DAE objective, and MultiUN as the parallel data for the XAE objective. In pre-training, they used Adam optimizer with a linear warm-up over the first $4k$ steps and linear decay for later steps, and the learning rate is set to $10^{- 4}$. The pre-training batch size is $64$, and the sequence length is set to $256$.
For fine-tuning on downstream NLG tasks, they used Adam optimizer with a learning rate of $5 \times 10^{- 6}$. They set the batch size as $16$ and $32$ for question generation and abstractive summarization, respectively. They truncate the input sentences to the first $256$ tokens. During decoding, they used beam search with a beam size of $3$, and limit the length of the target sequence to $80$ tokens.
The following table shows the evaluation results of monolingual supervised question generation for English and Chinese. BL is short for BLEU, MTR for METEOR, and RG for ROUGE.
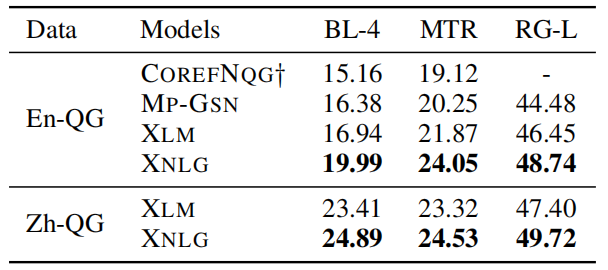
The following table shows the evaluation results of zero-shot Chinese-Chinese question generation. These results show that XNLG consistently performs better than baselines in both zero-shot and monolingual supervised setting.
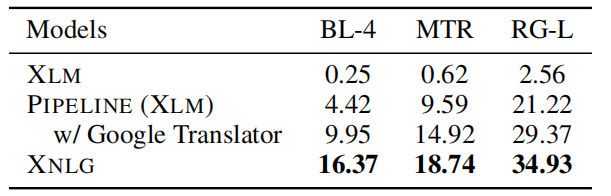
The following table shows the ROUGE evaluation results of supervised monolingual summarization. These results show that XNLG outperforms all the baseline models on both French and Chinese AS:
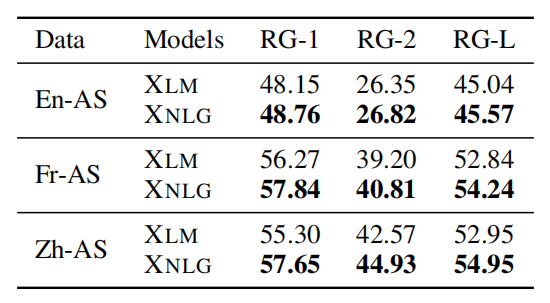
To check the effect of pre-training techniques on the performance, they conduct ablation studies models were evaluated on zero-shot Chinese-Chinese question generation task. The results show that the model benefits from the DAE objective for the zero-shot Chinese question generation task. The results also demonstrate that combining DAE and XAE can alleviate the spurious correlation issue and improves cross-lingual NLG.
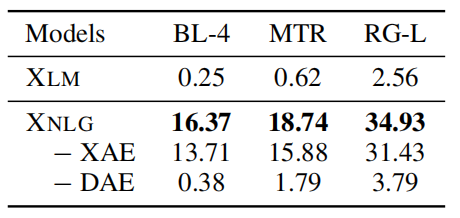